The Difference Between Big Data and Traditional Data
What are the benefits of big data? What is the difference between pure storage and traditional data? Let's find out more about The Difference Between Big Data and Traditional Data.
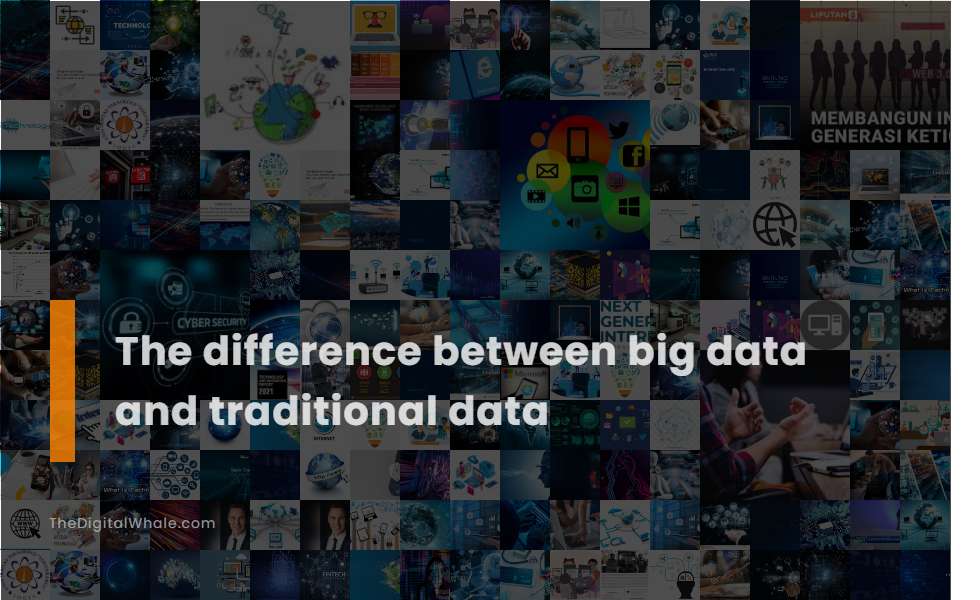
Data Size: Big data involves massive datasets (PB, ZB, EB), while traditional data is much smaller (GB, TB).
In the realm of data management, a significant distinction exists between Traditional Data and Big Data. Traditional data typically involves smaller-scale datasets, often measured in gigabytes or terabytes, and can be stored in centralized systems. In contrast, Big Data comprises massive datasets that reach the magnitude of petabytes, zettabytes, or even exabytes, necessitating advanced, large-capacity storage solutions. For a deeper understanding of these differences, you can explore the insights provided by H2K Infosys, which offer a detailed exploration of data storage challenges and methodologies associated with both traditional and big data platforms.
Data Structure: Traditional data is highly structured, while big data can be structured, semi-structured, or unstructured.
Traditional data is highly structured, organized in predefined formats like tables and columns, making it orderly and easy to analyze. In contrast, Big Data presents a more complex spectrum as it encompasses structured, semi-structured, or unstructured forms, which include a diverse range of data types such as text, images, and videos. This diversity allows for greater insights and applications but also introduces challenges in processing and managing vast volumes of information. For more insights on the differences between these forms of data, visit the GeeksForGeeks website.
Data Sources: Traditional data comes from centralized sources like ERP and CRM systems, while big data comes from distributed sources like social media, IoT devices, and sensors.
Traditional data is primarily sourced from centralized systems like ERP and CRM, where data is typically structured and comes from controlled and predictable environments such as transactional systems and enterprise applications. This is in contrast to big data, which arises from distributed and diverse sources including social media, IoT devices, sensors, and multimedia content. These sources contribute to the vast and varied nature of big data, which is often unstructured and generated at a high velocity. To explore more about the difference between traditional data and big data, it's essential to understand how each type fits into the broader landscape of data management and analytics. These distinctions impact how organizations leverage data for insights and decision-making.
Data Velocity: Traditional data is static or updated periodically, while big data is constantly changing and updated in real-time or near real-time.
Traditional data typically remains static and is updated on a periodic schedule, such as daily, weekly, or monthly. In contrast, Big Data is dynamic, constantly changing, and requires processing and analysis in real-time or near real-time. This fundamental difference highlights the need for distinct approaches in handling data in modern computational environments, where the ability to quickly analyze and respond to new information can provide significant advantages.
Data Variety: Traditional data is organized in predefined fields, while big data includes text, images, videos, and other diverse data types.
Traditional data is typically structured, organized in predefined fields such as tables, columns, and rows, and is commonly found in forms like spreadsheets and databases. In contrast, big data encompasses a more diverse range of formats, including structured, semi-structured, and unstructured data types. This can be anything from social media posts, images, videos, to sensor readings, and requires unique analytical approaches to handle its vast volume and variety. As discussed on Big Data Analytics, while traditional data analytics focuses on the structured data stored in relational databases, big data analytics is designed to manage and interpret massive, complex datasets across different formats, providing invaluable insights in today's data-driven world.
Related:
What are the benefits of cloud computing and its advantages? What is the best cloud computing platform for small businesses? Let's find out more about What Is the Cloud and How Can It Benefit Businesses?.
Infrastructure: Traditional data uses centralized database architectures, while big data requires distributed computing and cloud-based storage solutions.
Traditional data is managed using centralized database architectures, which are cost-effective and secure for smaller, structured data sets. In contrast, big data requires distributed architectures that link multiple servers or computers over a network, allowing for horizontal scaling and leveraging commodity hardware to reduce costs. For an in-depth comparison, you can explore more on Big Data vs Traditional Data where the complexities and infrastructures required for efficiently managing vast and diverse datasets are thoroughly examined.
Analysis: Traditional data is analyzed using SQL and statistical methods, while big data requires advanced analytics and specialized tools.
Traditional data analysis typically uses SQL and statistical methods to glean insights, while the advent of big data has introduced a necessity for advanced analytics. This includes incorporating machine learning and the use of specialized tools such as Hadoop, Spark, and NoSQL databases to effectively manage the intricate nature of big data. The complexity, volume, and variety of big data demand these sophisticated approaches, as traditional methods fall short when handling its dynamic and comprehensive nature. For more insights into these differences, GeeksforGeeks provides a detailed comparison of traditional data versus big data analytics.
Applications: Traditional data is ideal for routine business operations and historical trend analysis, while big data is used for uncovering hidden patterns, predicting trends, and gaining deep insights into customer behavior.
Traditional data is ideal for routine business operations, historical trend analysis, and standard reporting. In contrast, Big Data is used for advanced analytics, predictive modeling, real-time monitoring, and uncovering hidden patterns and deep insights into customer behavior and market trends. To explore more about these differences, visit the comprehensive guide on Big Data and Traditional Data on the Lise Du Network website.
Processing Power: Traditional data relies on conventional databases, while big data harnesses distributed computing and advanced algorithms.
Traditional data relies on conventional databases for processing, whereas big data utilizes distributed computing and advanced algorithms to handle the vast volume, variety, and velocity of data. For those looking to understand the differences in more detail, AlmaBetter offers a detailed comparison in their article on Big Data vs Traditional Data, highlighting the transformative impact of modern technologies on data management practices.
Complexity: Traditional data is relatively simple to manage and analyze, while big data is complex and requires specialized tools and techniques.
Traditional data is relatively simple to manage and analyze, whereas Big Data is complex and requires specialized tools and techniques due to its large volume, variety, and velocity. The need for advanced analytics like machine learning and data mining makes handling Big Data even more challenging. For more information on this topic, you can visit GeeksforGeeks, where they provide an in-depth comparison between traditional data and Big Data.
Related:
What are the latest technology trends? What are the latest technology trends? Let's find out more about The Best Ways To Stay Current with Technology.
Business Value: Traditional data provides insights into historical trends, while big data uncovers new opportunities and helps in making more informed decisions.
Traditional data provides insights into historical trends and past events, offering a lens into patterns and occurrences from previous periods. On the other hand, Big Data uncovers new opportunities and enables real-time analytics, facilitating future-oriented business decisions by analyzing a vast array of structured, semi-structured, and unstructured data. To understand these distinctions further, visit the comprehensive comparison at H2K Infosys. By leveraging the power of big data, organizations can access a broader spectrum of analytics, ultimately aiding in more informed decision-making processes.
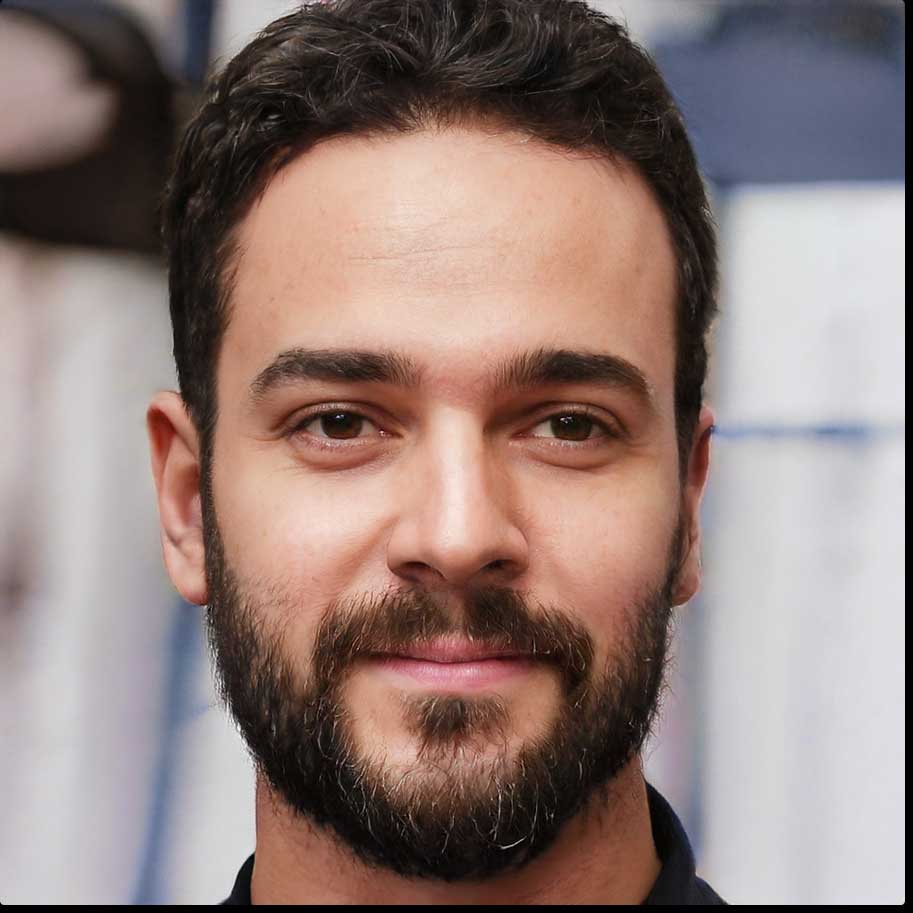